Annotation Statistics Tab#
The Statistics tab allows you to check on several aspects of the dataset and training results. You can find information about a ratio of all unannotated media items vs. annotated media items (images, videos and video frames) that are in the project. The tab allows you to monitor the accuracy score of the active model and see how many annotations exist for each class. You can also inspect the imbalance of object sizes (height and width) of each class.
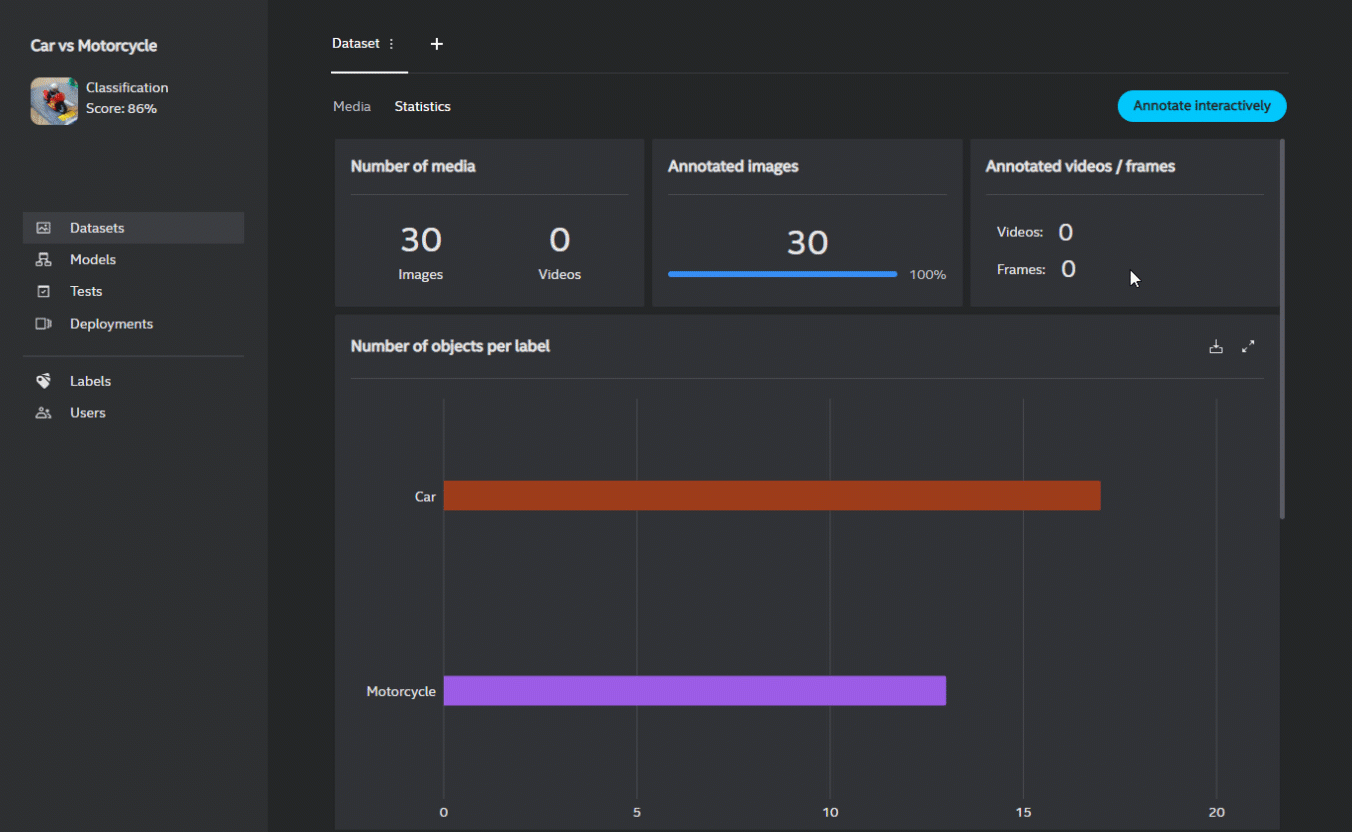
For anomaly projects, you will be presented with two accuracy scores:
Classification/Detection/Segmentation accuracy - this score pertains to accuracy on images i.e. it detects whether there is any anomaly in images
Localization accuracy - this score pertains to accuracy on objects i.e. it detects what regions in images contain anomalous objects
AI assistant score#
The AI assistant score is an accuracy measurement of the active learning model (AI assistant). The assistant performs predictions after annotating a required number of media items. The higher the score, the more precise AI assistant’s predictions will be. To increase the score, you need to annotate media items from the Active set (set by default). As you annotate more and more items, the score may keep increasing.
Number of objects per label#
These statistics present a number of annotated objects you added to your labels. The number of annotations may be higher than the number of media items in your dataset since in tasks such as object detection and image segmentation you will most likely annotate more than one object in an image or video frame. In a classification task, you can expect one label per media item.
The statistics are also presented in a graph to show you how your annotated objects stack up against all your created labels. The uneven representation may be an indicator of an imbalanced dataset which means that the model may predict certain objects with a higher precision than the other object. For example, if you have 10 annotations for a cat and 100 annotations for a dog, the model will be biased towards predicting dogs.
Object size distribution#
Object size distribution statistics allow you to inspect the imbalance of object sizes (height and width) of each class. The imbalance degree is calculated on the mean value of the object size aspect ratio per class.
When a model’s accuracy suddenly drops, you can investigate the reason behind the drop through the object size distribution graph. You can see the degree of too-tall, too-wide, and far-from-mean objects. If some objects deviate far from the mean, it may mean that you need more annotated objects of this size or to investigate further.
You can change the class by clicking on the menu and selecting the class of interest. After the selection, you will see the total number of objects in the center and the number of indicators of classified objects in the circle graph circumscribed with a color that will tell you if the objects are balanced, wide, or tall. Hovering over the color indicator displays the % and number of objects for each distribution. In the plot graph, you will see the distribution of the near-mean and far-from-mean of the objects via the near-mean ellipse, which is made using mean value and standard deviation.